Minisymposium Presentation
Scientific Machine Learning to Optimize Plasma Turbulence Simulations
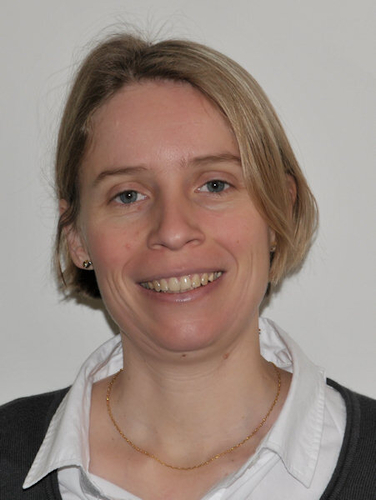
Presenter
Virginie Grandgirard received the PhD degree in mathematics and applications from Besancon University, France, in 1999. She then obtained the Habilitation à Diriger des Recherches in 2016. She is presently research director at CEA, France. She is the lead developer of the 5D non-linear gyrokinetic semi-Lagrangian code GYSELA used for plasma turbulence simulations https://gyselax.github.io/ . This code is highly parallelized up to hundreds of thousands cores. Her research interests focus on numerical methods for Vlasov equations, high performance computing and tokamak plasma turbulence and more recently on Physic Informed Neural Network. She has co-authored 60 publications in peer-reviewed journals.
Description
Controlled fusion offers the promise of sustainable and safe energy production on Earth. In magnetic fusion devices, the power gain increases nonlinearly with the energy confinement time. The quality of the plasma energy confinement thus largely determines the size and therefore the cost of a fusion reactor. Unfortunately, small-scale turbulence limits the quality of confinement in most fusion devices. Hence modelling of turbulent transport is mandatory to find routes towards improved confinement regimes. Numerical simulations are based on a kinetic description of the plasma that can only be performed on most powerful supercomputers. The gyrokinetic GYSELA code runs efficiently on several hundred thousand CPU cores. With a consumption of 150 million CPU hours per year, the code makes massive use of petascale computing capacities and manipulates Petabytes of data. However, there are still many challenges to overcome in order to achieve a full description of electron dynamics that will require exascale simulations. In this context, scientific machine learning could play an important role to optimize the storage and the number of CPU hours consumed. We will present here our on-going work on integrating in-situ diagnostics using Artificial Intelligence techniques for data compression and automatic detection of anomalies or rare events.