P18 - Exact Conservation Laws for Neural Network Integrators of Dynamical Systems
Description
We consider the construction of neural network surrogates for the solution of differential equations that describe the time evolution of physical systems. In contrast to other problems that are tackled by machine learning, in this case usually a lot is known about the system at hand: for many dynamical systems physical quantities such as (angular) momentum and energy are conserved. Learning these fundamental conservation laws from data is inefficient and will only lead to the approximate conservation of these quantities. We describe an alternative approach for incorporating inductive biases into the surrogate model. For this we use Noether's Theorem which relates conservation laws to continuous symmetries of the system and we incorporate the relevant symmetries into the architecture of the neural network Hamiltionian. We demonstrate that this leads to the exact conservation of (angular) momentum for a range of model systems that include the motion of a particle under Newtonian gravity, orbits in the Schwarzschild metric and two interacting particles in four dimensions. Our numerical results show that the solution conserves the relevant quantities exactly, is more accurate and does not suffer from instabilities that arise when using naive neural network surrogates.
Presenter(s)
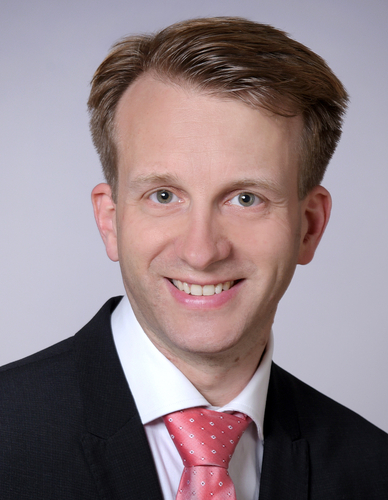
Presenter
Eike Mueller is an Associate Professor (Reader) in Scientific Computing at the University of Bath. His interests lie in efficient solvers for PDEs in atmospheric fluid dynamics, Multilevel Monte Carlo Methods and Machine Learning for differential equations. Eike's work on multigrid solvers has led to improvements to the operational Climate- and Weather forecast models used by the UK Met Office. Before joining the University of Bath, Eike obtained a PhD in Computational Particle Physics from the University of Edinburgh and worked on the parallelisation of numerical models for the transport and spread of atmospheric pollutants at the Met Office.