P20 - Fast Inference of Cosmology from High Resolution Maps Using Deep Learning
Description
Ongoing galaxy surveys like the Dark Energy Survey are designed to observe the large-scale structure of the Universe using a number of cosmological probes, such as weak gravitational lensing and galaxy clustering. Conventionally, constraints on the cosmological parameters are calculated by comparing two-point functions of the observables with semi-analytical theory predictions. However, we know that these physical fields contain information beyond what the two-point functions can capture as the Universe has evolved into nonlinear structures. With this project, we propose to leverage the expressive power of deep learning to extract this additional cosmological information by instead learning the summary statistic. To achieve fast progress in the scientific analysis, we aim to train the deep networks in under 24 hours. We present a GPU framework for fast and efficient analysis of cosmological maps on the A100 GPU nodes of the Perlmutter cluster at NERSC. We benchmark the pipeline for data parallel training on multiple A100 GPUs, both on a single and multiple Perlmutter nodes. We compare different distribution strategies, supervised and self-supervised loss functions, and graph convolutional and vision transformer network architectures on the sphere.
Presenter(s)
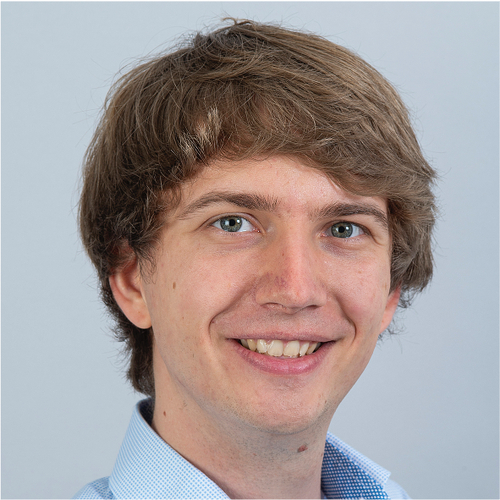
Presenter
I am a PhD student in the Cosmology Research Group at ETH Zurich working at the intersection of physical cosmology and machine learning. Specifically, I am interested in simulation-based inference and how the expressive power of deep learning can be leveraged in that context to extract more information from cosmological observations than traditional analysis methods permit. This overarching theme connects projects involving various data modalities, including images on the celestial sphere and point clouds, as well as different cosmological probes such as weak gravitational lensing and galaxy clustering.